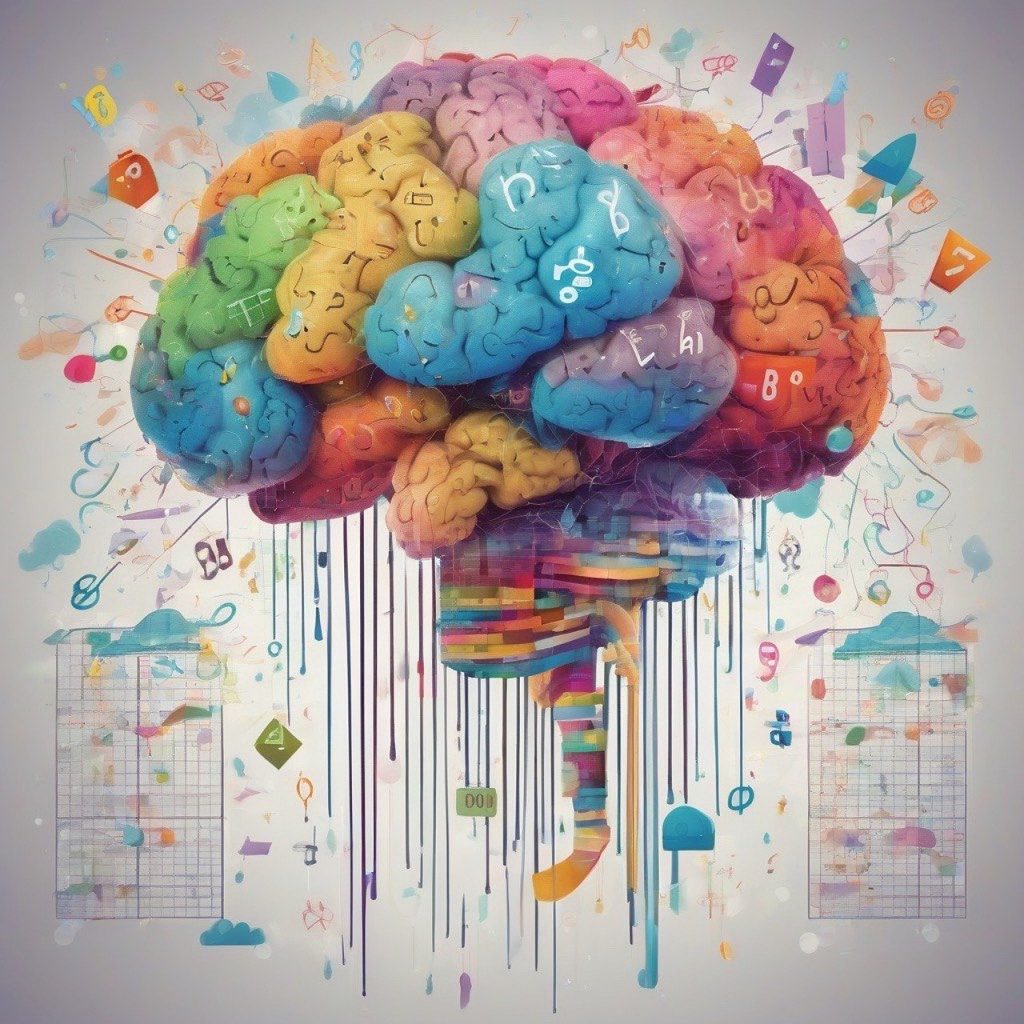
In today’s data-driven world, the ability to predict future outcomes with accuracy and confidence is invaluable for businesses, organizations, and individuals alike. Predictive analytics, the science of extracting insights from historical data to forecast future trends and behaviors, has long been a cornerstone of decision-making processes across various industries.
However, with the exponential growth of data and the complexity of modern challenges, traditional approaches to predictive analytics often fall short in capturing the intricacies of real-world phenomena.
Enter artificial intelligence (AI), a revolutionary paradigm that promises to transform predictive analytics by leveraging advanced algorithms and computational power to uncover hidden patterns, detect subtle correlations, and generate accurate predictions. The marriage of AI and predictive analytics represents a seismic shift in how we harness data to anticipate future events and make informed decisions.
The Emergence of AI in Predictive Analytics
As data volumes soar and computational capabilities expand, the traditional statistical methods that once dominated predictive analytics are giving way to AI-driven approaches. AI techniques such as machine learning, deep learning, and natural language processing have emerged as powerful tools for extracting insights from data and making accurate predictions.
One of the key advantages of AI in predictive analytics lies in its ability to handle complex and unstructured data with remarkable efficiency. Traditional statistical methods often struggle to cope with the diverse array of data sources available today, including text, images, and sensor data.
AI algorithms, on the other hand, excel at extracting meaningful patterns from these disparate sources, enabling organizations to derive insights from data that would have been previously untapped.
Key Components of AI-driven Predictive Analytics
- Data Acquisition and Preprocessing: Collection of relevant data from various sources, including structured and unstructured sources. Cleaning and preprocessing of data to ensure accuracy, consistency, and completeness. Transformation of raw data into a format suitable for analysis, including feature extraction and normalization.
- Feature Selection and Engineering: Identification of relevant features that contribute to predictive accuracy. Utilization of techniques such as feature scaling, dimensionality reduction, and feature extraction to enhance model performance. Incorporation of domain knowledge and expertise to engineer informative features that capture underlying patterns in the data.
- Model Selection and Training: Exploration of diverse AI algorithms and models, including regression, classification, clustering, and deep learning. Evaluation of model performance using metrics such as accuracy, precision, recall, and F1-score. Iterative refinement of models through techniques such as hyperparameter tuning, cross-validation, and ensemble methods.
- Evaluation and Validation Techniques: Validation of model performance on independent test datasets to assess generalization capabilities. Detection and mitigation of overfitting through techniques such as regularization and early stopping. Interpretation of model outputs and analysis of prediction errors to gain insights into model behavior and identify areas for improvement.
AI-driven predictive analytics relies on a synergy of these key components to generate actionable insights and drive informed decision-making. Leveraging advanced algorithms and computational techniques, organizations can unlock the full potential of their data assets and gain a competitive edge in today’s fast-paced business landscape.
Challenges and Considerations
Data quality and availability are paramount in predictive analytics, necessitating robust cleaning and preprocessing techniques to address issues like missing data and inconsistencies. Limited access to quality data and organizational data silos can hinder model development.
Interpretability of AI models poses a challenge due to their “black box” nature, necessitating transparency methods to understand model decisions. Ethical concerns arise regarding fairness and privacy, requiring governance frameworks and compliance with regulations like GDPR.
Overfitting, where models capture noise instead of patterns, and balancing complexity with generalization are key technical challenges. Regularization and validation strategies mitigate overfitting while simplifying models.
Addressing these challenges ensures responsible AI deployment, fostering innovation while upholding ethical standards and data privacy. A holistic approach integrating technical expertise with ethical considerations is essential for maximizing AI’s benefits while mitigating risks.
Best Practices for Implementing AI in Predictive Analytics
- Establish Clear Goals and Objectives: Define specific objectives and key performance indicators (KPIs) aligned with organizational priorities. Ensure stakeholders have a clear understanding of the intended outcomes and how predictive analytics will drive value.
- Build Interdisciplinary Teams: Form cross-functional teams comprising data scientists, domain experts, and business stakeholders. Foster collaboration and knowledge-sharing to leverage diverse perspectives and domain expertise.
- Utilize Scalable Infrastructure and Technologies: Invest in scalable computing infrastructure and cloud-based platforms to support AI model development and deployment. Leverage technologies such as distributed computing and containerization to streamline model deployment and scalability.
- Continuous Monitoring and Model Refinement: Implement robust monitoring systems to track model performance and detect deviations. Establish feedback loops for continuous learning and refinement of predictive models based on real-world feedback.
Future Directions and Trends
The evolution of AI in predictive analytics continues to unfold, shaping the future of decision-making and data-driven innovation. Integration with emerging technologies such as the Internet of Things (IoT) and blockchain promises to expand the scope and capabilities of predictive models, enabling more comprehensive and real-time insights.
Advances in automated machine learning (AutoML) and model interpretability are driving democratization, making predictive analytics more accessible to non-experts and enhancing trust in AI-driven decisions. Ethical considerations and regulatory frameworks are also evolving, highlighting the importance of responsible AI deployment and ensuring fairness, transparency, and accountability.
Conclusion
The fusion of AI with predictive analytics represents a paradigm shift in how organizations leverage data to anticipate future trends and make informed decisions. By harnessing the power of advanced algorithms and computational techniques, businesses across industries can unlock new opportunities for innovation, optimization, and growth.
However, this journey is not without its challenges, from ensuring data quality and interpretability to addressing ethical and privacy concerns. Nevertheless, by adhering to best practices, fostering interdisciplinary collaboration, and embracing emerging trends, organizations can navigate these challenges and reap the rewards of AI-driven predictive analytics.
As we look to the future, the possibilities are boundless, with AI poised to revolutionize decision-making processes, drive operational efficiencies, and create value on a scale previously unimaginable. By embracing AI responsibly and harnessing its transformative potential, organizations can chart a course towards a future where data-driven insights drive success and prosperity.